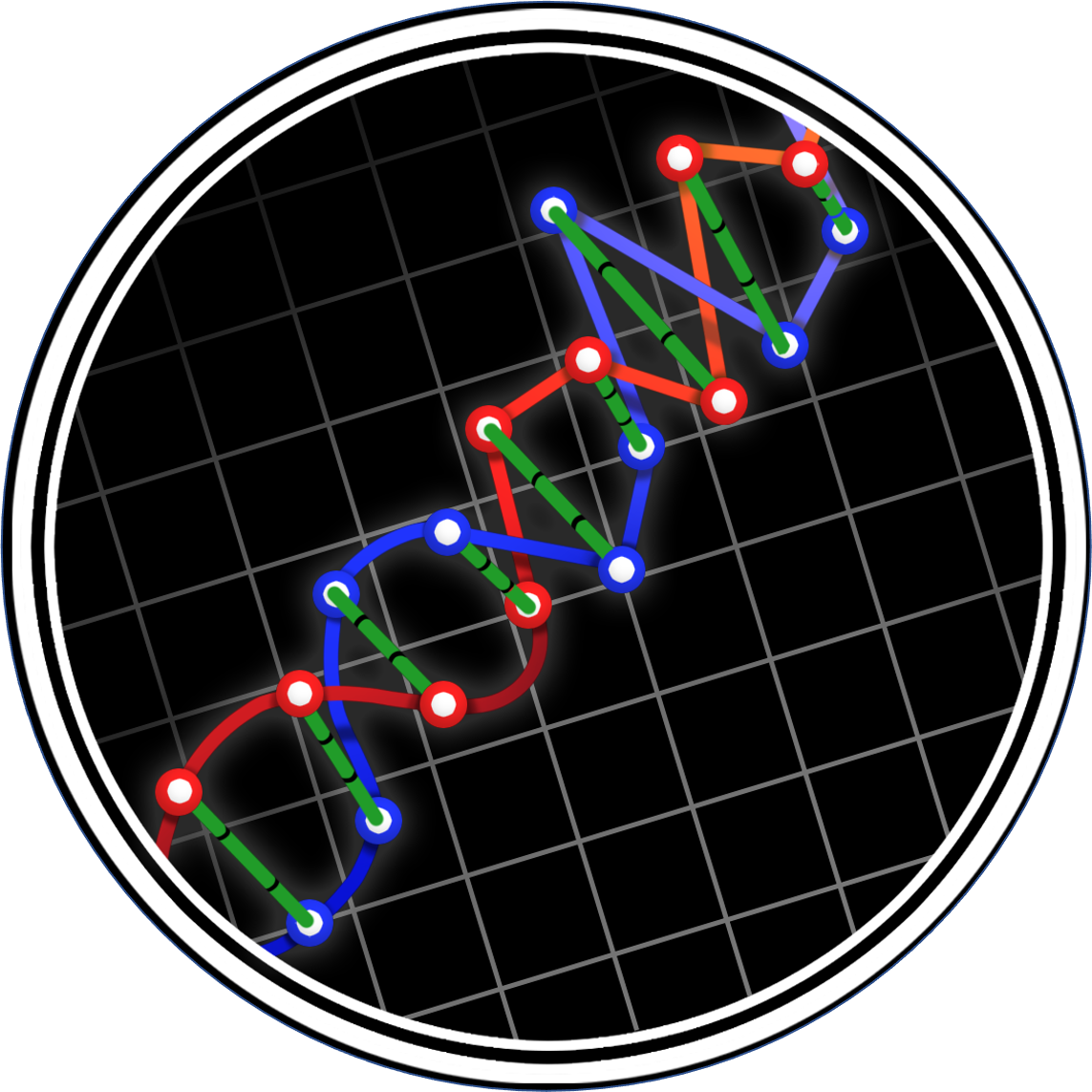
Data and Science with Glen Wright Colopy is a podcast covering critical scientific reasoning, particularly from a data science / machine learning / statistics perspective. Episodes typically focus on understanding of how to be better scientists and critical thinkers for the practical purpose of being a better data scientists. Previously called: ”Pod of Asclepius”
Episodes
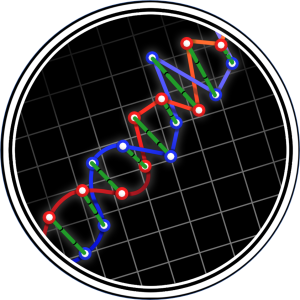
Tuesday Feb 22, 2022
Chris Tosh | The piranha problem in statistics
Tuesday Feb 22, 2022
Tuesday Feb 22, 2022
The piranha problem (too many large, independent effect sizes influence the same outcome) has received some attention on Andrew Gelman’s blog. But now it’s a paper! Chris Tosh (Memorial Sloan Kettering) talks about multiple views of the piranha problem and detecting the implausible scientific claims that are published. The butterfly effect makes an appearance.
If you enjoyed the science-vs-pseudoscience topics, you’ll enjoy this one.
0:00 - Coming up in the episode
2:35 - What is the Piranha Problem?
19:54 - Confusing effect sizes
23:11 - The "words & walking speed" study
26:22 - Declaration of independent variables
30:58 - Piranha theorems for correlations
37:07 - Piranha theorems for linear regression
40:37 - Piranha Theorems for mutual information
44:13 - Bounds on the independence of the covariates
46:12 - Applying the piranha theorem to real data
50:12 - Applying the piranha theorem across studies
54:05 - A Bayesian detour
1:00:12 - The butterfly effect & chaos
1:04:26 - Applying the piranha theorem to cancer research
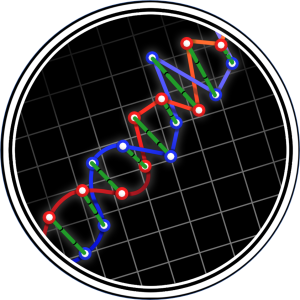
Tuesday Feb 08, 2022
Chris Holmes | AI, Digital Health, & The Alan Turing Institute
Tuesday Feb 08, 2022
Tuesday Feb 08, 2022
Chris Holmes is Professor of Biostatistics at the University of Oxford and Programme Director for Health and Medical Sciences at The Alan Turing Institute. Chris’ research interests include Bayesian nonparametrics (which is the right kind of nonparametrics), statistical machine learning, genomics, and genetic epidemiology.
0:00 - Intro
1:38 - Chris Holmes, Professor of Biostatistics at Oxford University
3:28 - UK Biobank & designing a valuable dataset
8:42 - Healthcare charities in the UK
11:16 - Digital Health: prioritizing research questions
19:55 - Bayes, nonparametrics, and Bayesian nonparametrics
23:30 - Model prediction is at the heart of Bayesian inference
28:00 - Prioritization in model building for biology
33:09 - Model constraints to generate valid inference
37:34 - Hypothesis driven science in statistical learning versus deep learning
43:30 - Developing models in genomics & clinical informatics
48:37 - Building stable, generalizable and robust models
52:41 - Important questions to think about
54:05 - Causal reasoning and clinical risk prediction
57:50 - What topic should the statistical community debate?
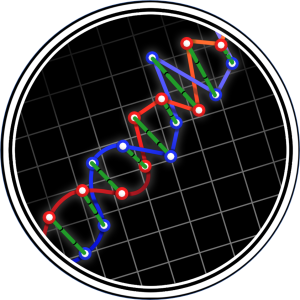
Thursday Feb 03, 2022
Thursday Feb 03, 2022
Philosophy of Data Science Series
Keynote with Deborah Mayo
Episode 1: Revolutions, Reforms, and Severe Testing in Statistical Thinking
In the first keynote of the Philosophy of Data Science Series we have a 2-part interview with Deborah Mayo (Virginia Tech).
In the first part of our keynote with Deborah Mayo we cover...
- The role of scientific revolution and its implications for statistics and data scientist.
- The necessity of statistical reforms and why philosophy will play a role.
- The value of severe testing of scientific claims.
Watch it on...
YouTube: https://youtu.be/S4VAEShM3BU
Podbean:
You can join our mail list at: https://www.podofasclepius.com/mail-list
We're always happy to hear your feedback and ideas - just post it in the YouTube comment section to start a conversation.
Thank you for your time and support of the series!
Topics:
0:00 - Preface to First Keynote Interview
2:00 - Welcome Deborah Mayo!
5:05 - What is the Philosophy of Statistics?
8:15 - What does philosophy add to data science?
16:10 - Scientific revolution in statistics
20:10 - Statistical reforms
24:25 - Replication & hypothesis pre-specification
31:00 - Failure is severe testing
37:25 - Error statistics
48:00 - Scientific progress and closing remarks
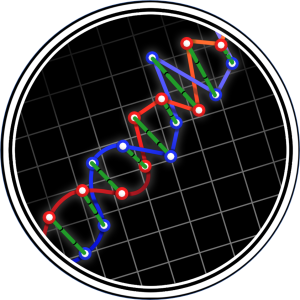
Tuesday Feb 01, 2022
Charlotte Deane | Bioinformatics, Deepmind’s AlphaFold 2, and Llamas
Tuesday Feb 01, 2022
Tuesday Feb 01, 2022
Charlotte Deane | Bioinformatics, Deepmind's AlphaFold 2, and Llamas
#datascience #ai
Charlotte Deane (Oxford University) talks about statistical approaches to bioinformatics, the evolution of Google Deepmind's AlphaFold 2 & its place in protein informatics deep learning landscape. She also describes humanizing antibodies, and the increasing role of software engineers in statistical research groups. The topic of llamas, camels, and alpacas (and their unique place in proteomics research) makes a surprise visit.
[Note: This episode was originally published in January 2022, but the file contained a buffering error, which prevented the full interview from being played. This version, published Feb 1, 2022 contains the full interview.]
Topics
0:00 Intro / An important topic to debate
3:50 What is a protein? Why are proteins foundational?
13:32 Immunotherapies, humanizing antibodies, & creating an scientific databases
16:04 Translating in silico research into immunotherapies
21:03 Nanobodies, camels, alpacas, & llamas.
25:05:00 Databases and data knowledge bases
33:21:00 Targeted therapies
39:45:00 Statistical modeling in proteomics
45:40:00 DeepMind AlphaFold's evolution
55:28:00 Software engineers in academic research groups
1:03:21 The adventure of science
1:07:42 Oxford Blues hockey & scientific debate
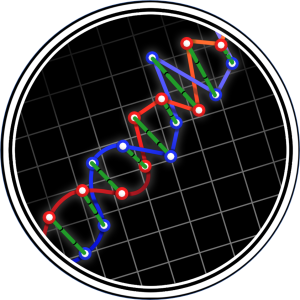
Wednesday Dec 01, 2021
Wednesday Dec 01, 2021
The philosophical community continuously aims to reconcile differing views on first person data and the consciousness of the mind. Is it possible to live without consciousness? Can one conceive thoughts without matching images to them? In this episode, Eric Schwitzgebel of the University of California tries to dissect such topics and questions to help us better understand the philosophical world.
Keywords: philosophy, epistemic data, first person data, stimulus error, imageless thought, consciousness
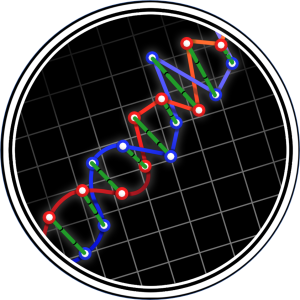
Monday Nov 22, 2021
Starting a Statistics Consultancy | Janet Wittes
Monday Nov 22, 2021
Monday Nov 22, 2021
Starting a Statistics Consultancy | Janet Wittes
The following interview was a keynote fireside chat with Janet Wittes (Statistics Collaborative, Inc.) titled "Statisticians as Entrepreneurs". It was recorded for the BBSW 2021 Conference (Nov 3 - 5 in Foster City, CA).
References:
BBSW 2021 Conference: https://www.bbsw.org/bbsw2021
Topics:
0:00 Janet's background prior to founding Statistics Collaborative, Inc.
3:00 Janet's initial research interest as a consultant
4:10 Why did Janet start her own business as opposed to joining a company or university.
5:45 Who were Janet's first clients?
8:00 What did Janet want to instill in her company?
15:50 Earning enough money to hire people
18:55 Initial ratio of clients to employees
22:42 Janet's company's statistical tech stack
25:00 Different challenges at different stages of the company
27:28 Growing a company but not taking on every possible client or project
28:13 Statisticians as entrepreneurs
37:00 Choosing the right people
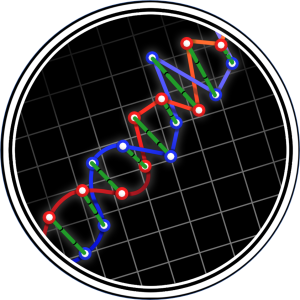
Tuesday Nov 16, 2021
Philosophy of Data Science | Jingyi Jessica Li | Advancing Statistical Genomics
Tuesday Nov 16, 2021
Tuesday Nov 16, 2021
Jingyi Jessica Li | Advancing Statistical Genomics
Jingyi Jessica Li (UCLA) describes common statistical pitfalls in genomic data analysis & the statistical reasoning required to correct these mistakes.
Common themes throughout include:
- Hypothesis-driven science & critical scientific reasoning over data
- p-values and non-sensical null hypotheses/distributions
- the value of appearing statistically rigorous
- researchers cutting intellectual corners & digging themselves into local minima
Episode Topics
0:00 A major advancement in genomic data leads to new statistical techniques
2:15 Hypothesis-driven science & hypothesis-free data analysis
2:55 A ChIP Seq Example
8:00 Misformulation of sampling variability
16:55 A false analogy: the permutation test
19:03 Losing my p-value religion: the value of statistical packaging
24:30 The Clipper Framework for false discovery rate control
31:50 Non-parametric developments
37:55 Inferred covariates
46:00 PseudotimeDE: inferences of differential gene expression along cell pseudotime
47:10 Selective inference
49:25 What biological/physiological data will be incorporated in the future?
52:30 Statistics, computer science, data science, ML, biology
57:05 Machine learning and prediction
1:01:30 Sophisticated models vs sophisticated research
1:07:45 Peer review in science
1:13:05 Hypothesis-driven science vs cutting intellectual corners
1:18:12 What topic should the statistics community debate?
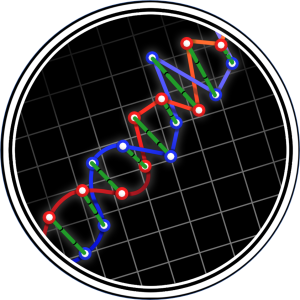
Tuesday Nov 09, 2021
Mine Çetinkaya-Rundel | Advancing Open Access Data Science Education
Tuesday Nov 09, 2021
Tuesday Nov 09, 2021
Mine Çetinkaya-Rundel | Advancing Open Access Data Science Education
#datascience #statistics #education
Mine Çetinkaya-Rundel (Duke University) describes the current and future states of statistics and data science education. Then she discusses the process of building open access learning material.
0:00 - Introduction
1:40 - Prioritizing topics in curricula
9:07 - Teaching with intent to test
11:22 - Statistics without computing
17:52 - What should be taught? How do we teach it?
19:07 - Computational thinking is valuable (to 31:45)
23:47 - Self reinforcing academics / positive feedback (to 31:45)
31:08 - Data science vs statistics (the computing angle)
37:55 - Statistical collaboration / technical collaboration
39:45 - Common language / imputation under ignorance
41:12 - Are some topics better for hands on or computational learning?
45:32 - Learning computation through visualization
52:40 - Video cut option before she gives an example
52:42 - Let them eat cake first.
56:08 - What is open source education? Open source vs open access.
59:36 - Advancing open source text books
1:03:55 - Economics of open source
1:07:55 - The open education ecosystem
1:12:17 - Modularizing & parallelizing learning topics
1:16:52 - Favorite dataset on OpenIntro.Org?
1:18:14 - What topic should the statistics community debate?
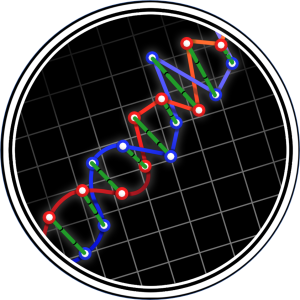
Sunday Sep 19, 2021
Sunday Sep 19, 2021
Jingyi Jessica Li | Statistical Hypothesis Testing versus Machine Learning Binary Classification
Jingyi Jessica Li (UCLA) discusses her paper "Statistical Hypothesis Testing versus Machine Learning Binary Classification". Jingyi noticed several high-impact cancer research papers using multiple hypothesis testing for binary classification problems. Concerned that these papers had no guarantee on their claimed false discovery rates, Jingyi wrote a perspective article about clarifying hypothesis testing and binary classification to scientists.
#datascience #science #statistics
0:00 – Intro
1:50 – Motivation for Jingyi's article
3:22 – Jingyi's four concepts under hypothesis testing and binary
classification
8:15 – Restatement of concepts
12:25 – Emulating methods from other publications
13:10 – Classification vs hypothesis test: features vs instances
21:55 - Single vs multiple instances
23:55 - Correlations vs causation
24:30 - Jingyi’s Second and Third Guidelines
30:35 - Jingyi’s Fourth Guideline
36:15 - Jingyi’s Fifth Guideline
39:15 – Logistic regression: An inference method & a classification method
42:15 – Utility for students
44:25 – Navigating the multiple comparisons problem (again!)
51:25 – Right side, show bio-arxiv paper
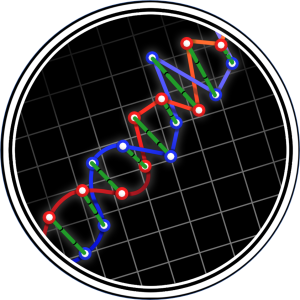
Sunday Aug 29, 2021
Sunday Aug 29, 2021
Gualtiero Piccinini | What Are First-Person Data?
First-person methods (and its associated data) have been scientifically and philosophically contentious. Are they pseudoscientific? Or simply pushing the bounds of scientific methodology? Obviously, I have no idea… so Prof. Gualtiero Piccinini (University of Missouri – St. Louis) provides a helpful introduction to the topic covering the key points of its history and the philosophical/scientific debate.
0:00 Why cover first-person methods & data?
2:26 First-person methods vs first-person data?
7:10 Are first-person data legitimate at all?
11:50 Phenomenology
13:26 First-person data is extracted from human behavior
18:25 Skepticism & arguments against first-person data
25:40 Psychophysics, introspectionists, behavioralists, cognitivists, and the origins of first-person data
35:20 Using new instruments & methods in science
46:00 Is this where the philosophers roam?
#datascience #statistics #science