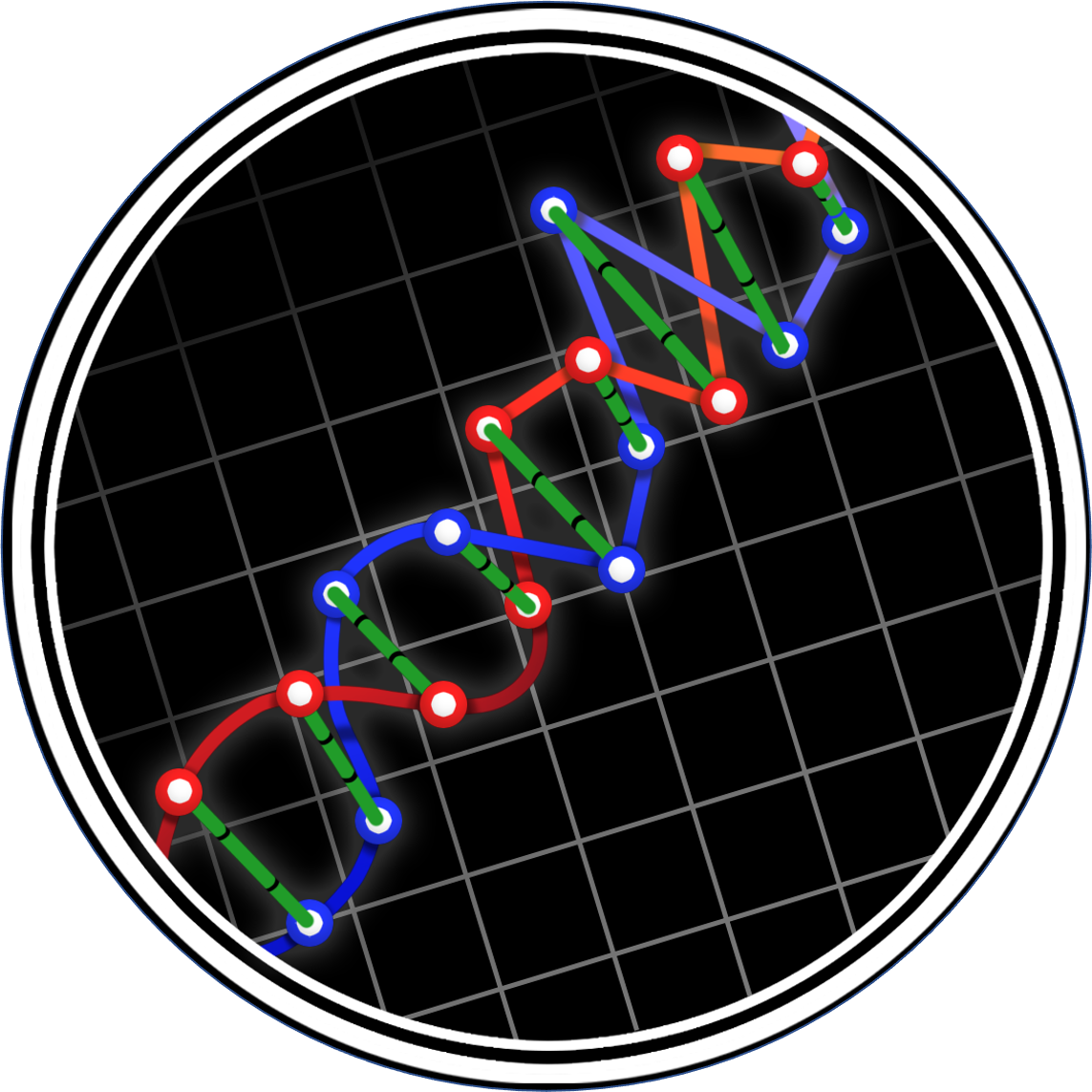
Data and Science with Glen Wright Colopy is a podcast covering critical scientific reasoning, particularly from a data science / machine learning / statistics perspective. Episodes typically focus on understanding of how to be better scientists and critical thinkers for the practical purpose of being a better data scientists. Previously called: ”Pod of Asclepius”
Episodes
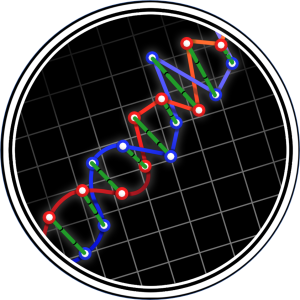
Monday Aug 16, 2021
David Dunson | Advancing Statistical Science | Philosophy of Data Science
Monday Aug 16, 2021
Monday Aug 16, 2021
David Dunson | Advancing Statistical Science | Philosophy of Data Science Series
A fundamental question in the philosophy of science is "what does it mean to make scientific progress?" We will have a series of episodes centered around this question for statistics and data science. In our first episode in the series, David Dunson (Duke University) discusses important advances in Bayesian analysis, big data, uncertainty, and scientific discovery.
Topic Timestamps
0:00 Intro to David Dunson
1:54 What does it mean to advance data science and statistics?
6:14 Industry & Optimization, Science & Uncertainty
8:14 Prediction & Discovery / Bayesian Modeling
14:13 What is “complex” data?
22:49 Big Data, Bayes, and Nonparametrics
33:50 Ad hoc approaches vs principled methods
37:08 Should Machine Learning Publications Refocus on Scientific Discovery?
39:50 Mathematically principled data science & statistics
51:40 Do Bayesians just use priors as regularizers?
55:16 Bayesian Priors and Tuning Inference Methods
1:00:00 Prioritize the Most Important Work in Data Science
1:07:07 Good Practices of Star Grad Students
1:13:17 The Science in Statistical *Science*
#datascience #science #statistics
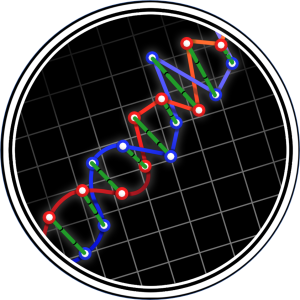
Monday Aug 02, 2021
Martin Kuldorff | Spatiotemporal Models of Disease Outbreaks
Monday Aug 02, 2021
Monday Aug 02, 2021
Note: This conversation was recorded June 25, 2021.
Martin Kuldorff | Spatiotemporal Models of Outbreaks
Martin Kuldorff (Harvard Medical School) talks about the integration of biological & demographic information (and general reality) in the spatiotemporal models used to detect disease outbreaks. He also discusses how these methods can be applied to non-infectious diseases like cancer.
0:00 - Spatio-temporal modeling of outbreaks
6:02 - Important features of spatio-temporal outbreak models
12:20 - Which diseases wouldn't you track for modeling?
19:02 - Multiple comparison adjustments of alarms
25:15 - Domain knowledge of outbreak features
29:30 Competing hazards & risks
34:30 Comparing hemispheres
37:00 - Bridging the gap for infectious diseases to cancer
45:10 - Retrospective data correction / changing monitoring
57:00 - Competing risks & statistics
1:01:30 - Deducing risks & affects through knowledge of immunological mechanisms
1:09:00 - Future scientific convos
#datascience #science
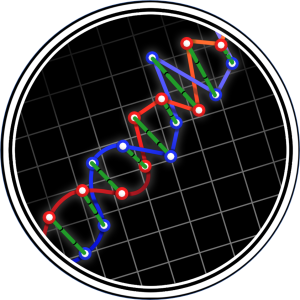
Monday Jul 19, 2021
Jason Costello | Data Science vs Software, Academia vs Industry
Monday Jul 19, 2021
Monday Jul 19, 2021
Interested in Data Science? Learn Data Science and Statistics from experts as they cover key topics in the field. The Data & Science podcast focusses on teaching data scientists how to think critically in order to solve data analysis problems across various scientific domains.
Jason Costello | Data Science vs Software, Academia vs Industry Jason Costello (Hypervector) describes his (non-trivial) transition from academic research into big tech and then the healthcare industry. He outlines a strategy to find the cool research problems that you get in academia while still delivering value to your company. We then talk about the interface of data science / machine learning and software.
0:00 Deploying Data Science into the Real World
8:24 Transitioning from Academic to Industrial Data Science
16:56 First step to delivering value to industry
21:38 Toy example of high value data science
25:28 Deep technical challenges are real and useful too!
29:59 Formalized logic in machine learning solutions
32:54 Data Science & Machine Learning Projects can fail.
38:50 Getting to the cool data science projects
47:21 Putting Machine Learning Models into Software
56:21 Software and Deduction, Machine Learning and Induction
1:06:06 Is Software A Deductive Complex System?
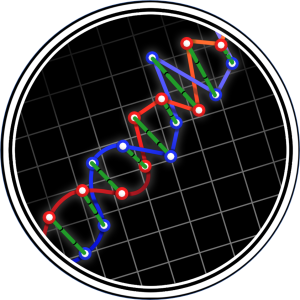
Monday Jun 14, 2021
Eric Daza | N-of-1 Science & Causal Inference | Philosophy of Data Science
Monday Jun 14, 2021
Monday Jun 14, 2021
Interesting in Data Science? Learn Data Science and Statistics from experts as they cover key topics in the field. The Data & Science podcast focusses on teaching data scientists how to think critically in order to solve data analysis problems across various scientific domains.
Eric Daza | N-of-1 Science & Causal Inference | Philosophy of Data Science
Much of our scientific inference revolves around the identification and replication of patterns in data. So what can be done when N=1? Eric Daza gives us a statistician's perspective on the ideas behind N-of-1 studies, its best examples, and strongest critiques.
0:00 - The purpose of N-of-1 & generalizability
3:30 - Successes and challenges in N-of-1
9:30 - A lightbulb moment
18:00 – Anomalies, Compliance, & Recurring Patterns
23:00 – Best Critiques of N-of-1, Safety, Efficacy
41:20 - Causal Inference
54:30 – Increasing the number of data scientists
1:03:30 – Biostatistics’ changing place in data science / statistical thinking
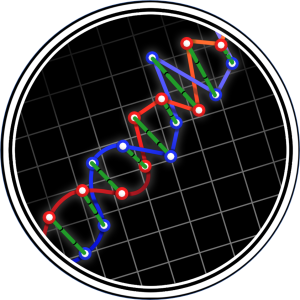
Tuesday Jun 01, 2021
Edward McFowland III | Anomalous Pattern Detection & Model Building
Tuesday Jun 01, 2021
Tuesday Jun 01, 2021
#datascience #statistics
Edward McFowland III | Anomalous Pattern Detection & Model Building
Edward McFowland III (Harvard Business School) describes the differences between "anomalies" and "anomalous patterns". Edward describes how this informs modeling strategies, in particular, when to use an off-the-shelf model versus building a bespoke model from scratch. He then covers how to draw inspiration from different scientific and technical fields.
0:00 Edward: Live in Conference
2:00 Outliers vs Anomalies vs Anomalous Patterns
9:30 Strategy to Identify Anomalous Data Patterns
19:15 Adding Complexity to Models
25:00 Building Blocks vs Comprehensive Models
39:05 New Pieces of Evidence
40:40 Deciding Data Science Strategies
52:30 Connecting the Technical Dots
58:40 Interdisciplinary Interests
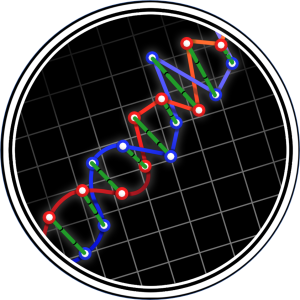
Wednesday May 26, 2021
Data Science Job Search | Advice + Q&A
Wednesday May 26, 2021
Wednesday May 26, 2021
#datascience #jobs #career #jobsearch #statistics
The Statistical Consulting Section of the ASA invited me to give a presentation on the data science job search followed by a Q&A.
They were kind enough to let me post it here (with minor edits).
My drawing of "cumulative cost" is wrong. It should intercept the "current cost" line at time = 0.
0:00 – Humility, Goals, & Human Data Points
5:00 – Play the Numbers Game
12:40 – Job vs Career
18:18 – Nonsensical Data Science Job Descriptions
25:40 – Technical Review & Presentation
30:00 – The Advantages of Early Career
37:25 – Save Job Descriptions / Industry vs Academia
46:10 – Career vs Job Clarification
53:10 – Bachelor’s vs Master’s vs Doctorate?
56:10 – Delivering Value Over Time
1:08:10 – Product vs Service
1:11:10 – Comments From an Academic Perspective
1:116:43 – Get Your Foot in the Door / Doing What You Love
1:25:50 – Future Q&A’s
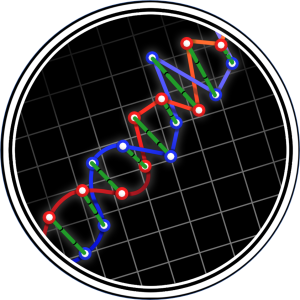
Wednesday May 19, 2021
Mike Evans | Statistical Reasoning & Evidence | Philosophy of Data Science Series
Wednesday May 19, 2021
Wednesday May 19, 2021
Mike Evans | Statistical Reasoning & Evidence | Philosophy of Data Science Series
Mike Evans (University of Toronto) describes his approach to statistical reasoning. Mike outlines how to recognize and address problems that are statistical in nature and why these approaches should be grounded in our ability to measure statistical evidence.
Watch it on YouTube at: https://youtu.be/Q7JpGZxHxXU
0:00 Statistical Reasoning
2:30 The Basic Problem: Reasoning on Statistical Problems
13:00 Rules of Statistical Inference
19:30 Bias (The Controversial Bit?!?!)
24:10 Steps of Statistical Reasoning
25:50 Connection to Philosophy of Science
27:35 Measuring Evidence (Frequentist vs Bayesian vs Loss Function)
29:49 Problems with the p-values
32:00 Choosing & Checking Priors
49:25 Idealism, Good Plans, Bad Plans
54:45 Describing Your Reasoning
59:20 Critiques of the Principle of Evidence
1:04:00 Data-Driven Science vs Hypothesis Driven Science
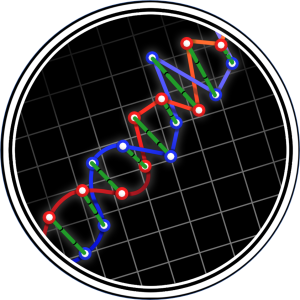
Thursday May 13, 2021
Deborah Mayo | Statistics & Severe Testing vs Pseudoscience
Thursday May 13, 2021
Thursday May 13, 2021
Deborah Mayo | Statistics & Severe Testing vs Pseudoscience
Watch it on… YouTube Podbean
In our fourth episode of the “science vs pseudoscience” mini-series, Deborah Mayo (Virginia Tech) specifies several necessary criteria to be scientifically rigorous. She gives several examples of how statistical thinking is essential to scientific thinking and why she believes that the “I’ll know it when I see it” approach to delineating science from pseudoscience is not a good approach.
Looking to catch up with the earlier “Science vs Pseudoscience” episode?
You can watch them here: Intro Episode 1 Episode 2 Episode 3
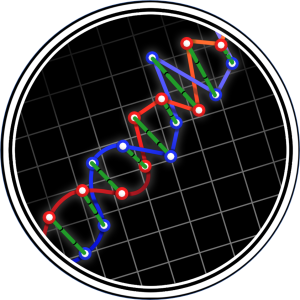
Monday May 10, 2021
Kristin Morgan | The Data Science of Sports Injury
Monday May 10, 2021
Monday May 10, 2021
Description: In the world of biomechanics, engineers continuously aim to innovate and create new models for better understanding of their research. In this episode, Kristin Morgan (University of Connecticut) returns to the show as she explains how they use gait as a form of diagnostic tool in maximizing human performance. Having experiences on sports herself, Morgan presents how they use gait to measure recovery from physical impairment, specifically for ACL-related injuries. Aside from this, however, she also explains how they use the same tool to measure recovery from cognitive impairment. An insightful episode for all!
Keywords: biomechanics, models, metrics, gait, engineering, statistics, cognitive impairment, physical impairment
0:00 - Intro
03:01 - Creating models for performance optimization
07:23 - Why gait is an effective diagnostic tool
11:38 - Maximizing gait in creating models for post-ACLR
17:35 - Manifestation of different injuries & models
22:01 - Modeling motor control
26:28 - Applying other models in biomechanics
30:50 - Using asymmetric walking for recovery
39:30 - Understanding cognitive impairment recovery
44:19 - Moving forward with gait as diagnostic tool
45:40 - Taking inspiration from other fields / Statistics in Engineering
47:45 - Engineering and statistics hand in hand
52:50 - Limitations of modeling in biomechanics
54:20 - Starting a career in biomechanics
58:20 - Including cognitive impairment
1:00:20 - Tailoring models to specific cases
1:05:33 - Applying the models to injuries other than ACL
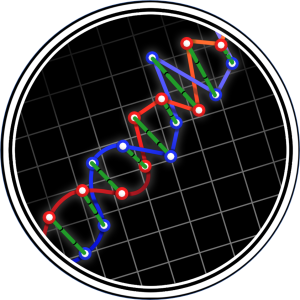
Wednesday May 05, 2021
Michael McRoberts | Football Analytics and Data-Driven Decisions
Wednesday May 05, 2021
Wednesday May 05, 2021
Michael McRoberts | Football Analytics and Data-Driven Decisions
Michael McRoberts (Championship Analytics Inc.) uses Monte Carlo simulations to provide strategy analytics to college and NFL football teams. Topics include communicating data-driven recommendations, the need to create counterfactual data, and asymmetric decision rewards.
0:00 The challenge of sports analytics
5:00 Analytics recommendations
16:00 Communicating data-driven recommendations
24:35 Vegas Odds & Ancillary Data
30:00 Football is way behind / Data science projects with a "runway"
41:25 Creating experiments and counterfactuals
49:30 Implementing data science insights
56:15 Asymmetric decision rewards
58:50 How to start in sports analytics
1:10:00 Data science vs analytics vs statistics