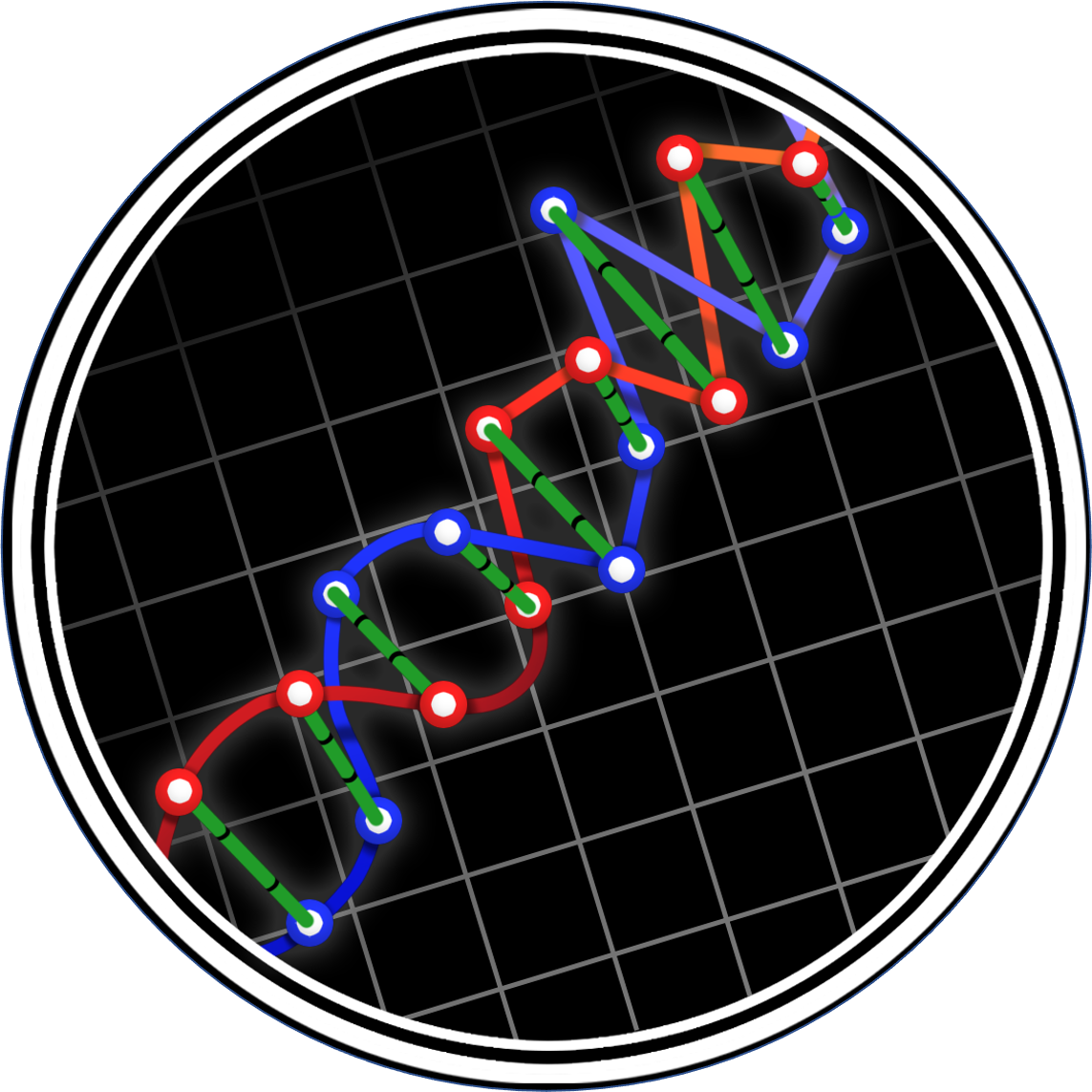
Data and Science with Glen Wright Colopy is a podcast covering critical scientific reasoning, particularly from a data science / machine learning / statistics perspective. Episodes typically focus on understanding of how to be better scientists and critical thinkers for the practical purpose of being a better data scientists. Previously called: ”Pod of Asclepius”
Episodes
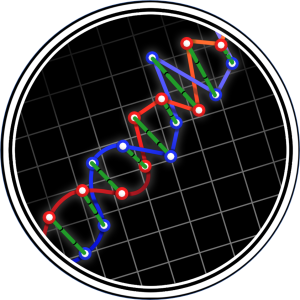
Friday Apr 30, 2021
Andrew Gelman & Megan Higgs | Statistics’ Role in Science and Pseudoscience
Friday Apr 30, 2021
Friday Apr 30, 2021
Andrew Gelman & Megan Higgs | Statistics' Role in Science and Pseudoscience
#datascience #statistics #science #pseudoscience
Our science vs pseudoscience discussion continues with Andrew Gelman (Columbia) and Megan Higgs (Critical Inference LLC). Andrew and Megan describe two critical roles that statistics plays in science.... but also how statistics can add the air of scientific rigor to bad research or help statisticians fool themselves. From there the conversation goes on in a way that only a conversation with Andrew and Megan can! A very fun episode.
0:00 - Two roles of statistics in science
4:50 - Many models were intended for designed experiments
10:30 - The biggest scientific error of the past 20 years
15:00 - Feedback loop of over-confidence / Armstrong Principle
21:00 - Science is personal
25:00 - The value of different approaches / Don Rubin Story
34:40 - Statistics is the science of defaults / engineering new methods
45:00 - The value of writing what you did
52:27 - Math vs science backgrounds + a thought experiment
1:01:20 - Fooling ourselves
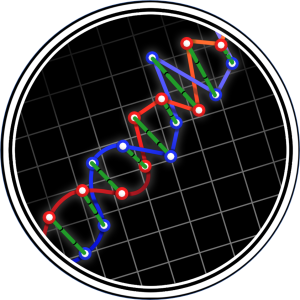
Tuesday Apr 27, 2021
Tuesday Apr 27, 2021
Irina Gaynanova (Texas A&M) describes why she thinks that replicability is a prerequisite for reproducibility in science and how scientists can (personally) start improving the replicability of research. We also discuss how the concepts of replicability/reproducibility can differ according to the domain-specific context and the methods used.
Please forward to any students or colleagues who would find this of interest!
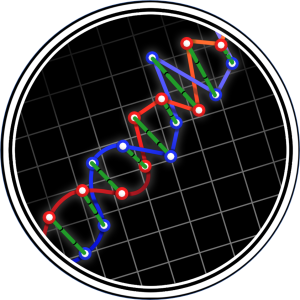
Monday Apr 19, 2021
Science vs Pseudoscience | Neil Manson | Philosophy of Data Science
Monday Apr 19, 2021
Monday Apr 19, 2021
#datascience #science #pseudoscience #criticalthinking #reasoning
We each like to think of ourself as scientific. I'm yet to meet someone who would embrace being called "pseudoscientific". But what makes the difference? In this episode, Neil Manson talks about the fallout from Thomas Kuhn's 1962 book "The Structure of Scientific Revolutions" and how this created a playbook for many modern critiques/attacks on scientific activity.
We have a new series that centers on the discussion of science vs. pseudoscience. Guests of different backgrounds share their insights on what really constitutes science and the highly-contested pseudoscience. The implications for data scientists and statisticians is very interesting, since many of the examples around this debate involved the conflicts between hypothesis-driven science vs data-driven science.
0:00 - Intro
0:43 - Science vs Pseudo/Bad/No Science
05:52 - Demarcation problem of science
12:07 - Incentives in science
13:00 - Glen forgets the word for "book"
13:40 - Luminiferous aether & lunch tables
18:19 - Keeping “good science” out of the science category
22:49 - Aiming to define science in relation to Kuhn’s theory
29:06 - Kuhn’s theory in action in various scenarios
32:53 - Logical fallacies in the world of science
46:17 - Intelligent design theory as science
51:14 - Distinction of different sciences
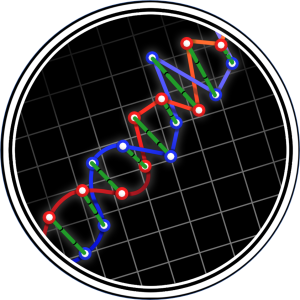
Wednesday Apr 07, 2021
Science vs Pseudoscience | Dien Ho | Philosophy of Data Science
Wednesday Apr 07, 2021
Wednesday Apr 07, 2021
We have a new series that centers on the discussion of science vs. pseudoscience. Guests of different backgrounds share their insights on what really constitutes science and the highly-contested pseudoscience. In today’s episode, we talk to Professor Dien Ho, PhD, a Professor of Philosophy and Healthcare Ethics, of the Massachusetts College of Pharmacy & Health Science University. Discover how philosophical ideas and theories are applied in hopes of understanding what really counts as science and what pseudoscience really is.
00:03 - Introductions
5:33 - What is pseudoscience?
08:53 - Legitimacy of other sciences
12:11 - What qualifies as science?
19:00 - Inductivism and empirical falsifiability
26:22 - Positivism and the importance of assumptions
31:36 - Assumptions and observations for data scientists
42:34 - The pursuit of science
49:17 - Scientism and revolutionary scientists
54:43 - Pinning down what science is
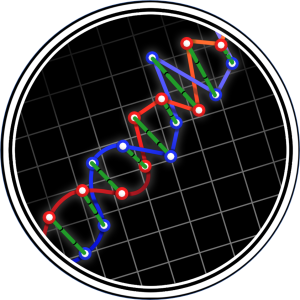
Wednesday Apr 07, 2021
New Science vs Pseudoscience Series (+ Renaming the Podcast)
Wednesday Apr 07, 2021
Wednesday Apr 07, 2021
We're launching a series on "Science vs Pseudoscience" tomorrow! Also we've rebranded to better reflect the focus of the podcast. The focus of the podcast isn't changing - it's still data science, critical scientistic reasoning, and figuring out how to figure stuff out!
Some fun reading on pseudoscience: https://philpapers.org/archive/MONP-1...
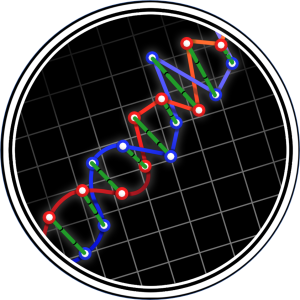
Thursday Mar 11, 2021
Environmental Data Science | Career Q&A
Thursday Mar 11, 2021
Thursday Mar 11, 2021
We've received a lot of questions from early career data scientists interested in starting a career in environmental science and climate science. Elizabeth Mannshardt (EPA), Grant Weller (Optum Labs), and Megan Higgs (Critical Inference LLC) sit down to give you your answers!
Thinking about a career change to Environmental Data Science? We invite you to listen to some career growth strategies and opportunities in environmental data science” podcast.
Throughout the episode we discuss how to transition from other careers to an environmental data scientist. How to get quantitative skills in order to switch to environmental science. Ways someone can learn environmental science and get an entry job as an environmental scientist. Plus, “in career growth should one focus on a specific domain or to go broad?”.
00:00:00 Start
00:03:14 Introduction
00:09:03 Transition from other fields to Environmental Scientist.
00:22:27 How to get quantitative skills in order to switch to environmental science.
00:36:20 In career growth should one focus on a specific domain or be broad.
00:44:00 Ways someone can learn environmental science.
00:48:49 Ways people can get an entry job as an environmental scientist
01:07:54 Final comments
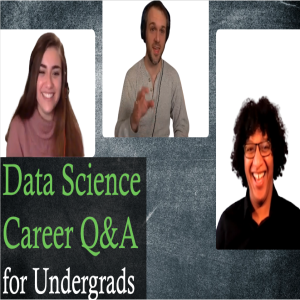
Thursday Feb 25, 2021
Data Science Career Q&A for Undergrads
Thursday Feb 25, 2021
Thursday Feb 25, 2021
#datascience #career #job
Data Science Career Q&A for Undergrads with Mallory LaRusso
We continue to answer data science career questions. We've heard back from a lot of different groups about the world of data science. In this episode, we're talking about undergraduate DS job prospects. Mallory LaRusso is a senior at NCSU finishing her BS in Statistics, and Minor in Genetics. Watch/Listen as Glen and Richard answer questions from our guest Malory as she tries to understand ways of how to properly transition from being an undergrad student to becoming a data scientist. From questions about data scientists’ typical workday to their most challenging projects to date, we’ve got it all covered in this episode!
Keywords: data analytics, data science, programming, coding, workday, work culture, educational background
0:00 - Introduction
02:20 - Series overview
06:44 - Educational and career path
09:16 - Typical work day
17:00 - The importance of writing in data science
20:39 - Work culture
23:30 - Type of data you work with
26:00 - Mathematical vs Statistical Models
31:45 - The harder DS jobs are what’s left
32:35 - Favorite project as data scientist
36:00 - Work on a real problem
39:55 - Data scientists’ degrees
44:15 - Difference of data analytics and data science
54:13 - Favorite programming language
1:01:29 - How data science jobs will change
1:07:42 - Largest data set to have worked with
1:17:02 - Advice for students to prepare for data science roles
1:36:30 - What advantage does an undergraduate have?
1:40:22 - Wrap-up
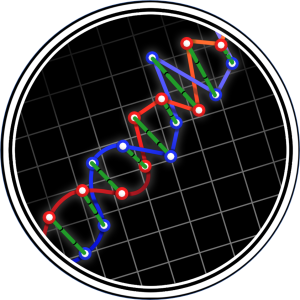
Tuesday Feb 16, 2021
Philosophy of Data Science | Step-change and Anomaly Detection | Alex Bolton
Tuesday Feb 16, 2021
Tuesday Feb 16, 2021
#datascience #ai #earlycareer
Philosophy of Data Science Series
Session 3: Data Science Highlight Reel
Episode 4: Alex Bolton on Step-change and Anomaly Detection
Who makes it into the highlight reel of data science? Alex Bolton for doing the hard work of analyzing data to figure out exactly when things don't look "normal". We discuss the critical reasoning behind step-change detection and anomaly/novelty detection. Alex provides several real-world examples of the data and challenges.
Watch it on...
YouTube: https://www.youtube.com/watch?v=097FO1JDkhU
Podbean:
We're always happy to hear your feedback and ideas - just post it in the YouTube comment section to start a conversation.
Thank you for your time and support of the series!
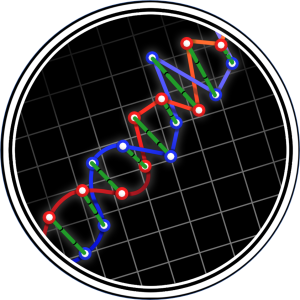
Monday Feb 08, 2021
Irina Gaynanova | Replicating Clinical Metrics & Innovating New Methods
Monday Feb 08, 2021
Monday Feb 08, 2021
Philosophy of Data Science Series
Session 3: Data Science Highlight Reel
Episode 2: Irina Gaynanova on Replicating Clinical Metrics & Innovating New Methods
Who makes it into the highlight reel of data science? Irina Gaynanova for her work on replicating clinical metrics for deployment. She then goes into how her grasp of the scientific domain helps her innovate new methods and metrics. Regardless of whether you work in the clinical domain, this is an example of rigorous scientific thinking in data science.
We're always happy to hear your feedback and ideas - just post it in the YouTube comment section to start a conversation.
Thank you for your time and support of the series!
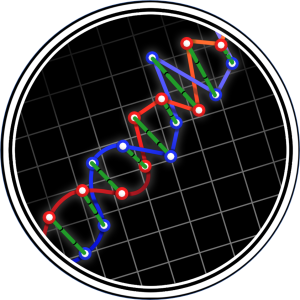
Thursday Jan 21, 2021
Karel Moons | Validating Medical Predictive Models | Philosophy of Data Science
Thursday Jan 21, 2021
Thursday Jan 21, 2021