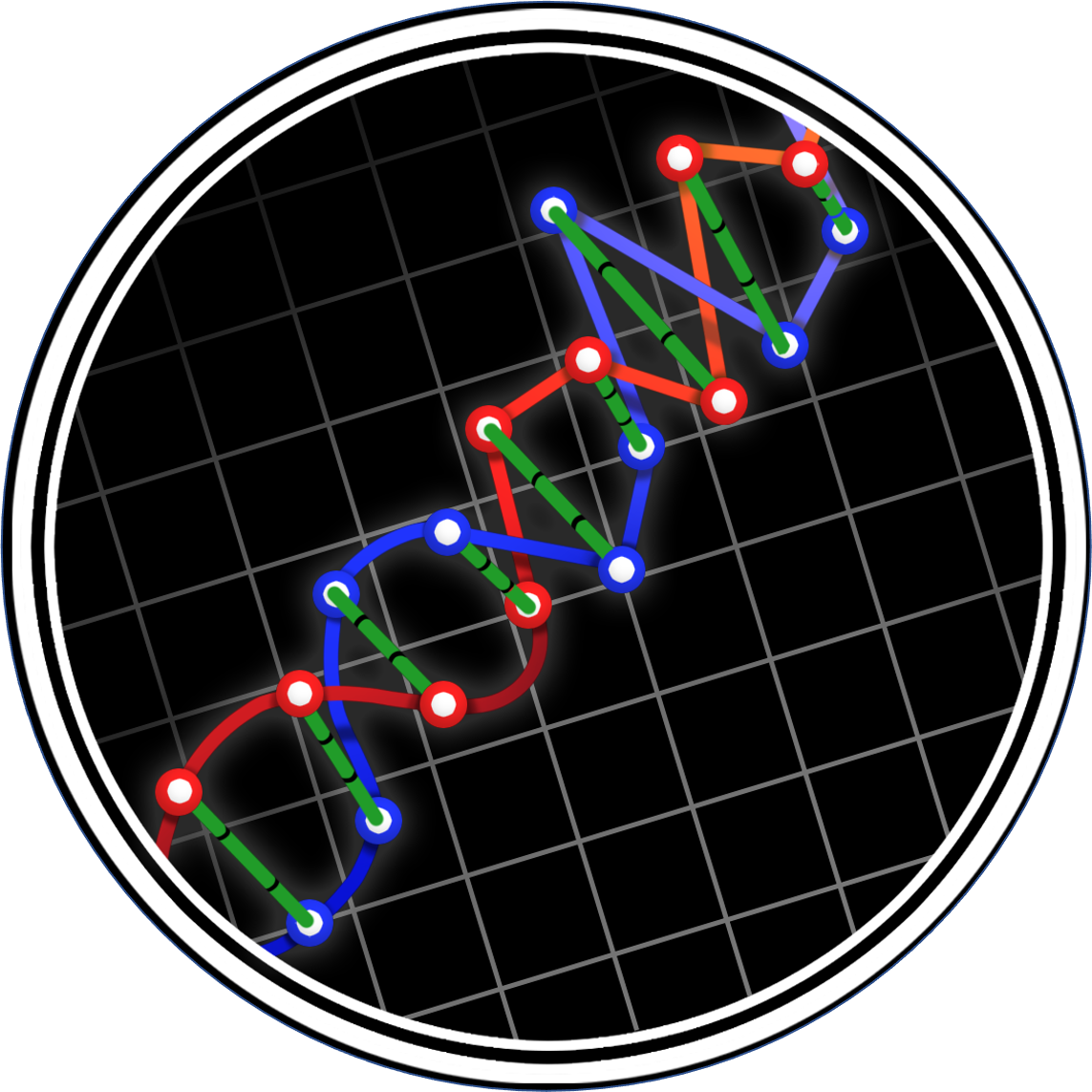
Data and Science with Glen Wright Colopy is a podcast covering critical scientific reasoning, particularly from a data science / machine learning / statistics perspective. Episodes typically focus on understanding of how to be better scientists and critical thinkers for the practical purpose of being a better data scientists. Previously called: ”Pod of Asclepius”
Episodes
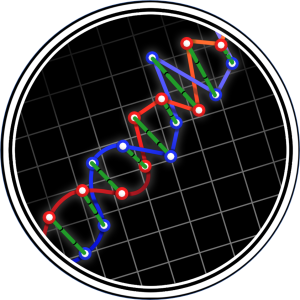
Tuesday Aug 02, 2022
Keith O’Rourke | The Logic of Statistics
Tuesday Aug 02, 2022
Tuesday Aug 02, 2022
Keith O'Rourke | The Logic of Statistics
Dr. Keith O'Rourke talks about the logical reasoning behind statistical modeling. Topics include mathematical vs scientific reasoning, whether science has become too stats focused, and vice versa.
Watch it on...
Youtube: https://youtu.be/FqE4ROHBKpY
Podbean: https://dataandsciencepodcast.podbean.com/e/keith-o-rourke-the-logic-of-statistics/
Topic List:
0:00 - The logic of statistics
0:30 - What is scientific statistics?
5:15 - The logic of statistics and CS Pierce
9:15 - Role of representation in statistics: explicit vs implicit
14:13 - Diagrammatic Reasoning
18:45 - Why is modeling counterfactual?
19:33 - How can statisticians become better scientists?
28:40 - Science is hard
31:24 - Computational approaches to learning
42:00 - Learning through metaphor
46:28 - Diagrammatic representations vs math
48:40 - Is science too statistics-focussed?
59:35 - Is statistics sufficiently science-focussed?
1:08:40 - Scientific Debate
#statistics #datascience #science
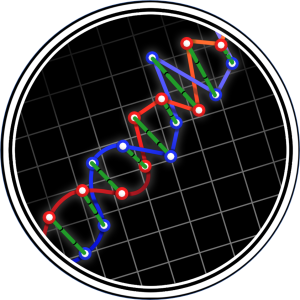
Monday Jul 25, 2022
Jack Fitzsimons | Evil Models: Hiding Malware in Neural Networks
Monday Jul 25, 2022
Monday Jul 25, 2022
Jack Fitzsimons | Evil Models: Hiding Malware in Neural Networks
Did you know that it's possible to hide malware in neural networks? Actually, you can hide malware in many statistical models. This is the subject of two recently-published papers (aptly titled "EvilModel" & "EvilModel 2.0"). Dr. Jack Fitzsimons makes it easy to understand how this is done, using techniques that began long before computers.
Watch or listen on...
Youtube: https://youtu.be/QBnk8ogL8Nk
Podbean: https://dataandsciencepodcast.podbean.com/e/jack-fitzsimons-evil-models-hiding-malware-in-neural-networks/
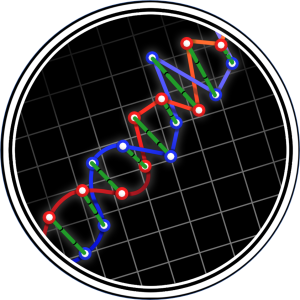
Sunday Jul 17, 2022
Scott Cunningham | Causal Inference (The Mixtape)
Sunday Jul 17, 2022
Sunday Jul 17, 2022
Scott Cunningham | Causal Inference (The Mixtape)
Scott Cunningham (Baylor University) discusses the ideas of his book "Causal Inference: The Mixtape". Topics include trusting inference in the absence of counterfactuals and the challenges of apply scientific methods to social phenomena.
Watch it on...
YouTube: https://youtu.be/yNaCudDVTkY
Podbean: https://dataandsciencepodcast.podbean.com/e/scott-cunningham-causal-inference-the-mixtape/
0:00 - COMING UP...
0:35 - What makes it into the mixed tape?
7:10 - Coding to learn
11:15 - More people are expected to work with data & code
12:50 - Design vs program vs estimators
20:40 - Causation with zero correlation
27:00 - Optimization make everything endogenous
28:45 - The hospital example
29:30 - Credible scientific discovery vs motivated discovery
39:55 - Different meanings of causality
43:30 - The impossible counterfactual
47:00 Counterfactual nihilism
49:20 Social experiments / Defund the police
53:35 - Skepticism about the science of social phenomena
1:05:20 - The Italian crime example
1:16:30 - Scientific debate
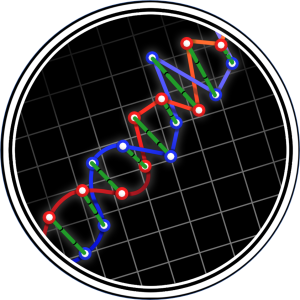
Sunday Jul 10, 2022
Eric Daza | Important Ideas in Causal Inference
Sunday Jul 10, 2022
Sunday Jul 10, 2022
Eric Daza | Important Ideas in Causal Inference
YouTube: https://youtu.be/K5nsSMJVIT0
Andrew Gelman and Aki Vehtari wrote a paper titled, "What are the most important statistical ideas of the past 50 years?". The first idea in the list is "counterfactual causal inference". Eric Daza (Evidation Health) walks us through the main ideas of the Gelman & Vehtari paper, drawing examples from several fields, including medical & healthcare statistics.
Topics
0:00 - Coming up...Correlation vs Causation
1:20 - Most important statistical ideas over the last 50 years
6:10 - Counterfactual Causal Inference
9:40 - Assumptions Change between Applied Domains
21:10 - Propensity Score Methods
25:15 - Transportability of Scientific Results
26:30 - People don't want generalizable results
32:00 - Generic Computation Algorithms
37:00 - Reweighting
43:57 - Matching Methods
58:20 - Medical Data is Higher Dimensional that we think.
1:00:15 - Is a Trial Population Representative?
1:10:35 - Causal Models in the Future
1:18:45 - Apostates Welcome
1:21:45 - Scientific Debate
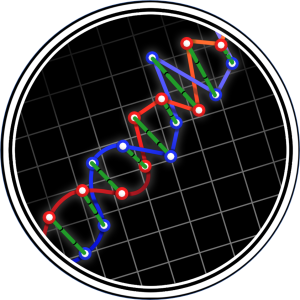
Monday May 09, 2022
Wenting Cheng & Weidong Zhang | Advances in Biotech/Biopharma
Monday May 09, 2022
Monday May 09, 2022
Wenting and Weidong discuss how the statistical challenges in the biopharm industry have proliferated with the unique demands of biotech and related life science industries.
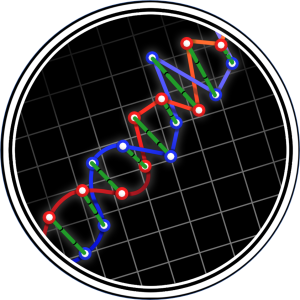
Monday May 09, 2022
Ruda Zhang | Gaussian Process Subspace Regression
Monday May 09, 2022
Monday May 09, 2022
Ruda Zhang | Gaussian Process Subspace Regression
Ruda Zhang (Duke University) walks us through "Gaussian Process Subspace Regression for Model Reduction" by Zhang, Mak, and Dunson.
To keep the topic interesting for both the early career & advanced audience we recap key points at a high level so that no one gets lost.
This episode involves a presentation, so you may prefer to watch the YouTube version here: https://youtu.be/IPtqUUG4XcY
Ruda's website: https://ruda.city/
The paper: https://arxiv.org/abs/2107.04668
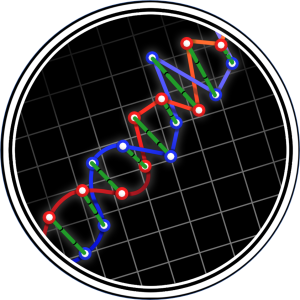
Wednesday Apr 13, 2022
Ruda Zhang | Math-Science Duality
Wednesday Apr 13, 2022
Wednesday Apr 13, 2022
Ruda Zhang | Math-Science Duality
Watch it on...
Youtube: https://youtu.be/GoDwen-RGZg
Podbean: https://dataandsciencepodcast.podbean.com/e/ruda-zhang-math-science-duality/
Statistics is thought to reside at the interface of science and mathematics. Ruda Zhang (Duke University) discusses the friction at this interface and the role that both mathematical formalism & observational/data-driven intuition play in scientific discovery. A great topic for anyone interested in statistics' role in scientific discovery.
#datascience #ai #science #mathematics
Topic List
00:00 COMING UP...
2:44 Ruda Zhang's compendium of cool ideas + a Gaussian process PSA
7:08 Is intuition undervalued in scientific research?
10:16 Mathematics vs observational science. Rigor vs intuition.
14:07 Intuition & discovery precedes mathematical rigor
21:58 Mathematics vs empirical science & the complexity of induction
30:24 Abstract thinking & the cost/benefit of discovery
37:25 The efficient frontier / Pareto Front of knowledge
42:55 Pragmatism and competence
50:24 Math /science dualism
1:15:52 AI making scientific discoveries
1:19:15 Statistical & scientific debate
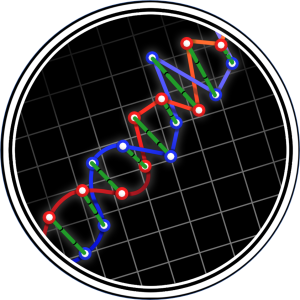
Tuesday Apr 05, 2022
Simon Mak | Integrating Science into Stats Models
Tuesday Apr 05, 2022
Tuesday Apr 05, 2022
Simon Mak | Integrating Science into Stats Models
#statistics #science #ai
It’s a common dictum that statisticians need to incorporate domain knowledge into their modeling and the interpretation of their results. But how deeply can scientific principles be embedded into statistical models? Prof. Simon Mak (Duke University) is pushing this idea to the limit by integrating fundamental physics, physiology, and biology into both the models and model inference. This includes Simon’s joint work with Profs. David Dunson and Ruda Zhang (also of Duke University).
Scientific reasoning AND stats. What more could we ask for?
Enjoy!
Watch it on....
YouTube: https://youtu.be/bUbZO7R4z40
Podbean: https://dataandsciencepodcast.podbean.com/e/simon-mak-integrating-science-into-stats-models/
00:00 - COMING UP….Scientists & Statisticians
02:09 - Introduction - Integrating scientific knowledge into AI/ML
06:08 - How much domain knowledge is sufficient?
09:15 - Choosing which prior knowledge to integrate into a model
14:49 - Black box & gray box optimization
19:50 - Non-physics examples of integrating scientific theory into ML models
22:45 - Scientific principles & modeling at different scales
27:20 - Correlation is one just way of modeling linkage
36:37 - Conditional independence & different-fidelity experiments
39:40 - Innovation vs incorporation of known information in the model
42:52 - Aortic stenosis example
52:49 - Which mathematics can be used to represent scientific knowledge
57:09 - How to acquire scientific domain knowledge
1:02:45 - Complementary approaches to integrating science
1:06:48 - Gaussian process & integrating priors over functions
1:12:48 - A topic for statisticians and scientists to debate:science-based vs data-based learning.
Simon Mak's Webpage: https://sites.google.com/view/simonmak/home
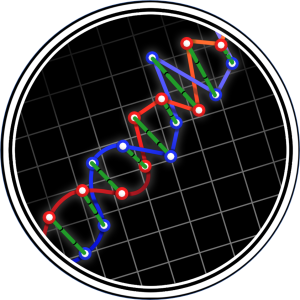
Wednesday Mar 16, 2022
Martin Goodson | Practical Data Science & The UK’s AI Roadmap
Wednesday Mar 16, 2022
Wednesday Mar 16, 2022
Martin Goodson | Practical Data Science & The UK's AI Roadmap
#ai #datascience #startups
Martin Goodson (Evolution AI) describes the key aspects of the UK's AI Roadmap & responses to the document by members of the Royal Statistical Society. In particular, Martin describes the disconnect between the priorities of AI startups and industry practitioners on one side, and government and academia on the other. Martin also outlines which skills early career data scientists should focus on while in school versus after entering the workforce.
Also available on....
YouTube: https://youtu.be/T9qRl6Hclhg
Topic List
0:00 COMING UP: Scientific culture & AI
1:25 The UK AI Roadmap
8:44 Who is a data science “practitioner”?
12:53 Data science in AI startups
20:36 Is there a disconnect between practitioners & academia?
25:09 Key skills for new data science graduates
32:03 Coding & production level data science
39:30 Learning the right data analysis skills at the course-level.
45:32 AI leadership
58:40 AI from academia & OpenSource initiatives
1:05:37 Large institutions' impact on the AI field
1:08:24 Back to the UK AI roadmap
1:12:16 Building an AI community
1:13:15 AI in our lifetime: Moonshots & realistic goals
1:14:31 Scientific debate
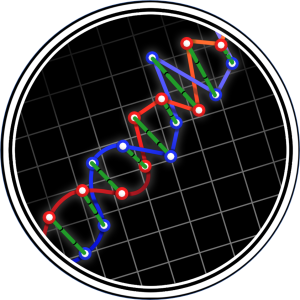
Monday Feb 28, 2022
Jack Fitzsimons | Data Security, Privacy, & Artificial Intelligence
Monday Feb 28, 2022
Monday Feb 28, 2022
Dr. Jack Fitzsimons (Oblivious AI) gives a high-level introduction to the technologies that can either exploit or protect your data privacy. If you'd like to survey the landscape of data privacy-preserving technologies (from someone who's building the tech) this is a good place to start!
#datascience #privacy #ai
0:00 - Coming up...
3:24 - Introduction
6:20 - Data privacy and privacy enhancing technologies
13:00 - History of privacy enhancing technologies
19:54 - Differential privacy: Hiding the influence of a single data point
22:52 - Trading data utility for data privacy
38:32 - Tracking algorithms and how they decide user preferences
42:04 - Preserving privacy: Anonymizing data & VPNs
50:17 - Exploration vs Exploitation: Combining best of multiple domains to tackle problems
54:13 - Federated learning, input and output privacy of data
58:45 - Balancing data privacy vs data-driven personalization
1:05:50 - What should data scientists/statisticians debate?